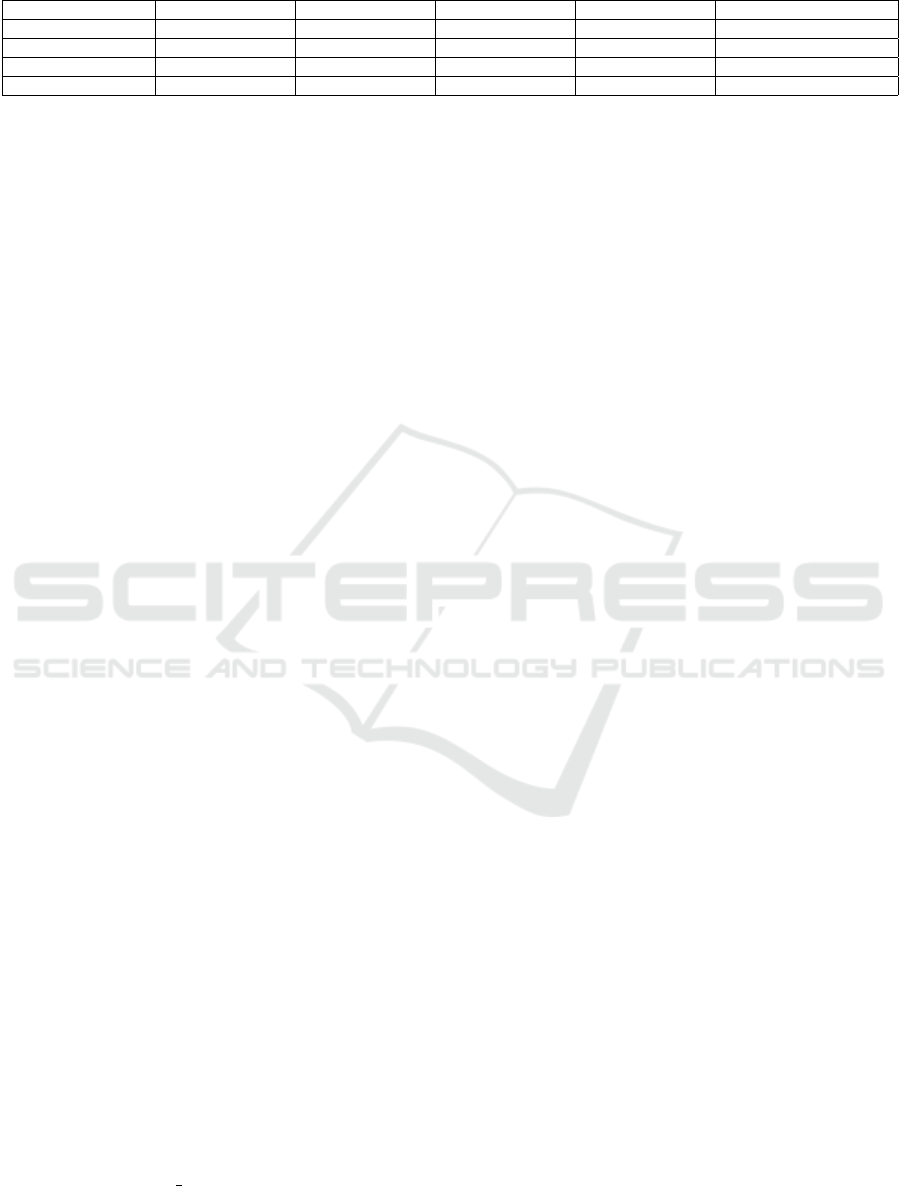
Table 2: Comparison of models with Weighted Average Accuracy.
Models Frame Set 1 (%) Frame Set 2 (%) Frame Set 3 (%) Frame Set 4 (%) Weighted Average (%)
AlexNet 80.17 85.18 57.92 81.78 79.04
LeNet 78.79 87.20 50 68 75.96
EfficientNet-B2 73.95 81.56 53.36 93.64 77.63
Swin Transformer 94.79 91.86 74.65 77.21 86.67
The performance of four models AlexNet, LeNet,
EfficientNet-B2, and Swin Transformer-Tiny was
compared against expert-labeled ground truth data.
Accuracy was evaluated for each model across four
frame sets, and a weighted average was calculated
to account for the changing number of frames in
each set. Swin Transformer-Tiny achieved the high-
est weighted average accuracy (86.67%), clearly
outperforming AlexNet (79.04%), EfficientNet-B2
(77.63%), and LeNet (75.96%). The accuracy of
all models across individual frame sets and their
weighted averages, showcasing the exceptional per-
formance of Swin Transformer-Tiny is shown in Ta-
ble 2
5 CONCLUSION
This paper introduces an intelligent pavement con-
dition rating system customized to cycling infras-
tructure, addressing the limits of traditional road-
focused assessment methods. The proposed CRSI
provides a scalable and comprehensive framework
using a dataset of over 40,000 annotated images,
this study evaluated state-of-the-art DL models, with
Swin Transformer-Tiny demonstrating superior per-
formance and robustness across various cycling con-
ditions. The framework offers a practical solution for
automated infrastructure evaluation, enabling data-
driven maintenance and increasing the safety and us-
ability of cycle routes. Future work will focus on ex-
panding the dataset and integrating real-time analysis
capabilities to optimize performance further. This re-
search sets a strong foundation for modernizing cy-
cling infrastructure assessments, supporting safer and
more sustainable transportation networks.
ACKNOWLEDGMENT
This research is conducted with the financial sup-
port of the EU Commission Recovery and Resilience
Facility under the Research Ireland OurTech Chal-
lenge Grant Number 22/NCF/OT/11220 and the sup-
port of Science Foundation Ireland under Grant num-
ber [SFI/12/RC/2289 P2] the Insight SFI Research
Centre for Data Analytics. The authors acknowl-
edge support from Transport Infrastructure Ireland
and Katleen Bell-Bonjean (Social Impact champion
from GORTCYCLETRAILS.ie). For the purpose
of open access, the author has applied for a CC
BY public copyright license to any author-accepted
manuscript version arising from this submission.
REFERENCES
Amr A. Elhadidy, S. M. E.-B. and Elbeltagi, E. E. (2021). A
simplified pavement condition index regression model
for pavement evaluation. International Journal of
Pavement Engineering, 22(5):643–652.
Aslan, O. D., Gultepe, E., Ramaji, I. J., and Kermanshachi,
S. (2019). Using artifical intelligence for automat-
ing pavement condition assessment. In International
Conference on Smart Infrastructure and Construction
2019 (ICSIC), pages 337–341.
ASTM International (2018). Standard practice for roads and
parking lots pavement condition index surveys. Re-
trieved from https://www.astm.org.
Gu, Y., Khojastehpour, M., Jia, X., and Han, L. D. (2024).
Estimating pavement condition by leveraging crowd-
sourced data. Remote Sensing, 16(12).
Ibragimov, E., Kim, Y., Lee, J. H., Cho, J., and Lee, J.-J.
(2024). Automated pavement condition index assess-
ment with deep learning and image analysis: An end-
to-end approach. Sensors, 24(7).
Krizhevsky, A., Sutskever, I., and Hinton, G. E. (2012).
Imagenet classification with deep convolutional neu-
ral networks. In Pereira, F., Burges, C., Bottou, L.,
and Weinberger, K., editors, Advances in Neural In-
formation Processing Systems, volume 25. Curran As-
sociates, Inc.
Kuznetsov, D. V., Bugakova, A. V., and Bereza, A. N.
(2024). Intelligent information system for monitoring
the road pavement conditions. In 2024 Conference of
Young Researchers in Electrical and Electronic Engi-
neering (ElCon), pages 208–211.
Lecun, Y., Bottou, L., Bengio, Y., and Haffner, P. (1998).
Gradient-based learning applied to document recogni-
tion. volume 86, pages 2278–2324.
Liu, Z., Lin, Y., Cao, Y., Hu, H., Wei, Y., Zhang, Z., Lin,
S., and Guo, B. (2021). Swin transformer: Hierarchi-
cal vision transformer using shifted windows. In Pro-
ceedings of the IEEE/CVF International Conference
on Computer Vision (ICCV), pages 10012–10022.
Lytton, R. F. (1987). Concepts of pavement performance
prediction and modeling. Transportation Research
Record, 1123:67–77.
VEHITS 2025 - 11th International Conference on Vehicle Technology and Intelligent Transport Systems
674