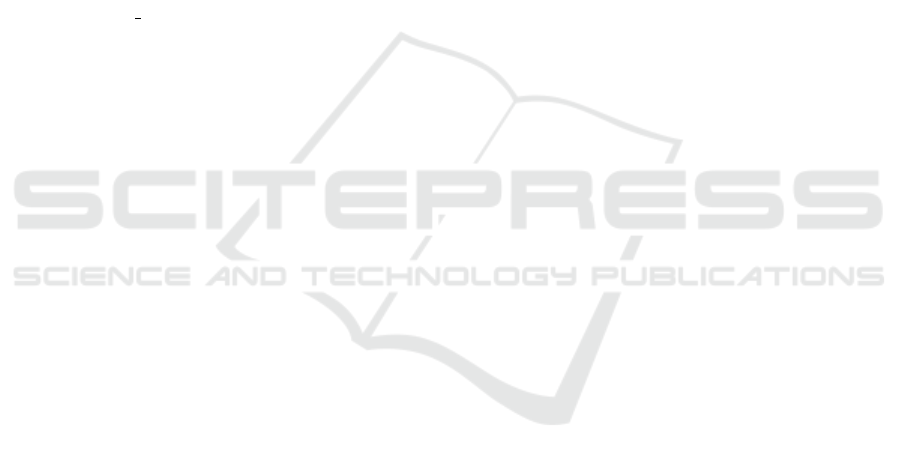
validating and refining the proposed system through
more field experiments, exploring correlations with
measurement devices such as profilers, and obtain-
ing a reliable pavement assessment measure such as
IRI while addressing implementation and continuing
with stakeholder collaboration. Adopting such sys-
tems can play a pivotal role in advancing sustainable
transportation by ensuring cycling tracks remain safe
and appealing to users.
ACKNOWLEDGMENTS
This research was conducted with financial support
from the EU Commission’s Recovery and Resilience
Facility through the Research Ireland OurTech
Challenge (Grant Number 22/NCF/OT/11220)
and Science Foundation Ireland (Grant Number
SFI/12/RC/2289 P2) under the Insight SFI Research
Centre for Data Analytics. The authors also acknowl-
edge the support of Transport Infrastructure Ireland
and Katleen Bell-Bonjean, Social Impact Champion
from GORTCYCLETRAILS.ie. For Open Access, a
CC BY public copyright license has been applied to
any Author Accepted Manuscript resulting from this
submission.
REFERENCES
Abed, A., Rahman, M., Thom, N., Hargreaves, D., Li, L.,
and Airey, G. (2023). Predicting pavement perfor-
mance using distress deterioration curves. Road Ma-
terials and Pavement Design.
Alatoom, Y. I. and Obaidat, T. I. (2022). Measurement of
street pavement roughness in urban areas using smart-
phone. International Journal of Pavement Research
and Technology, 15(5):1003–1020.
Baig, M. H., Ayala Garcia, J. A., Qureshi, W. S., and Ullah,
I. (2025). Towards assessing cycleway pavement sur-
face roughness using an action camera with imu and
gps.
Board, T. R., National Academies of Sciences, E., and
Medicine (2018). Inertial Profiler Certification for
Evaluation of International Roughness Index. The Na-
tional Academies Press, Washington, DC.
Federal Highway Administration (FHWA) (n.d.). Practical
guide for quality management of pavement condition
data collection. U.S. Department of Transportation.
GoPro (2024). Gpmf-parser: Parser for gpmf™ formatted
telemetry data used within gopro® cameras. GitHub
repository. Retrieved from GitHub.
Ibragimov, E., Kim, Y., Lee, J. H., Cho, J., and Lee, J.-J.
(2024). Automated pavement condition index assess-
ment with deep learning and image analysis: An end-
to-end approach. Sensors, 24(7):2333.
Jeong, J.-H. and Jo, H. (2024). Toward real-world
implementation of deep learning for smartphone-
crowdsourced pavement condition assessment. IEEE
Internet of Things Journal, 11(4):6328–6337.
Jeong, J.-H., Jo, H., and Ditzler, G. (2020). Con-
volutional neural networks for pavement roughness
assessment using calibration-free vehicle dynamics.
Computer-Aided Civil and Infrastructure Engineer-
ing, 35(11):1209–1229.
Liang, H., Pagano, R. G., Oddone, S., Cong, L., and
De Blasiis, M. R. (2024). Analysis of road surface
texture for asphalt pavement adhesion assessment us-
ing 3d laser technology. Remote Sensing, 16(11).
Loprencipe, G., de Almeida Filho, F. G. V., de Oliveira,
R. H., and Bruno, S. (2021). Validation of a low-cost
pavement monitoring inertial-based system for urban
road networks. Sensors, 21(9).
Massow, K., Maiwald, F., Thiele, M., Heimendahl, J.,
Protzmann, R., and Radusch, I. (2024). Crowd-based
road surface assessment using smartphones on bicy-
cles. In 2024 International Conference on Artificial
Intelligence, Computer, Data Sciences and Applica-
tions (ACDSA), pages 1–8, Victoria, Seychelles.
Nasrallah, A. A., Abdelfatah, M. A., Attia, M. I. E., and El-
Fiky, G. S. (2024). Positioning and detection of rigid
pavement cracks using gnss data and image process-
ing. Earth Science Informatics, 17:1799–1807.
Ozoglu, F. and G
¨
okg
¨
oz, T. (2023). Detection of road
potholes by applying convolutional neural network
method based on road vibration data. Sensors,
23(22):9023.
Perraton, D., Di Benedetto, H., Sauz
´
eat, C., Bankowski, W.,
Partl, M., and Grenfell, J. (2011). Rutting of bitumi-
nous mixtures: wheel tracking tests campaign analy-
sis. Materials and Structures, 44(5):969–986.
Shah, S. M. H., Qureshi, W. S., O’Dea, G., and Ullah, I.
(2025). Intelligent pavement condition rating system
for cycle routes and greenways.
Shin, S.-P., Kim, K., and Le, T. H. M. (2024). Feasi-
bility of advanced reflective cracking prediction and
detection for pavement management systems using
machine learning and image detection. Buildings,
14(6):1808.
Transport Infrastructure Ireland (2022). Rural cycleway de-
sign (offline & greenway) (dn-geo-03047). Transport
Infrastructure Ireland. Retrieved from Transport In-
frastructure Ireland.
Wen, W. (2008). Road roughness detection by analysing
imu data. Master’s thesis, School of Architecture and
the Built Environment, Royal Institute of Technology
(KTH), Stockholm, Sweden.
Youwai, S., Chaiyaphat, A., and Chaipetch, P. (2024).
Yolo9tr: A lightweight model for pavement damage
detection utilizing a generalized efficient layer aggre-
gation network and attention mechanism. Journal of
Real-Time Image Processing, 21(5).
VEHITS 2025 - 11th International Conference on Vehicle Technology and Intelligent Transport Systems
682