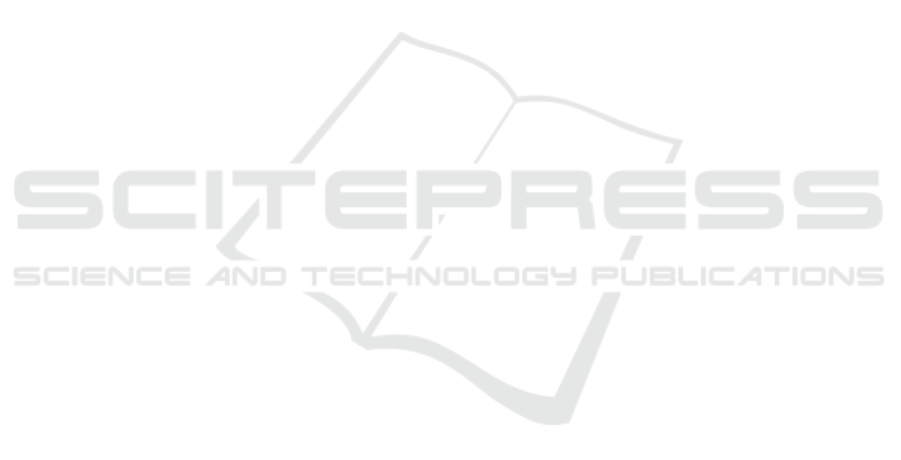
NLP techniques and ML models. Our methodology
offers a scalable and automated solution for patent
analysis through the integration of LLM-based struc-
tured data extraction, relevance categorization, and
impact calculation. The findings from our initial vali-
dation demonstrate that the methodology successfully
extracts significant insights from patents, presenting a
viable alternative to conventional review techniques.
The suggested methodology improves patent anal-
ysis by utilizing advanced LLMs to extract structured
information, categorizing patents according to their
technological relevance, and assessing their potential
impact through predictive modeling. Our research il-
lustrates that the integration of NLP approaches with
machine learning allows for a more thorough evalua-
tion of patents, aiding in the detection of significant
advances in the IoT sector.
Notwithstanding the encouraging outcomes, nu-
merous obstacles persist. The initial validation was
performed on a restricted dataset, and subsequent re-
search should aim to broaden the methodology to
encompass a wider and more varied collection of
patents. Furthermore, enhancing the effect estima-
tion model through advanced time-series forecasting
and the integration of external elements like market
adoption patterns could improve predictive accuracy.
A vital topic for enhancement is the augmentation
of the interpretability of AI-driven patent evaluation,
guaranteeing that the methodology yields practical in-
sights for academics, enterprises, and policymakers.
The next steps will focus on augmenting the
dataset, refining domain-specific LLM models for
patent analysis, and assessing the methodology across
several technological areas outside IoT. Additionally,
incorporating expert feedback into the review process
could enhance the trustworthiness of automated as-
sessments. This research addresses these problems,
enhancing AI-assisted intellectual property analysis
and facilitating more efficient, data-driven decision-
making in patent review.
REFERENCES
Abbas, A., Zhang, L., and Khan, S. U. (2014). A literature
review on the state-of-the-art in patent analysis.
Aristodemou, L. and Tietze, F. (2018). The state-of-the-art
on intellectual property analytics (ipa): A literature
review on artificial intelligence, machine learning and
deep learning methods for analysing intellectual prop-
erty (ip) data.
Casola, S. and Lavelli, A. (2022). Summarization, simplifi-
cation, and generation: The case of patents.
Chang, Y., Wang, X., Wang, J., Wu, Y., Yang, L., Zhu,
K., Chen, H., Yi, X., Wang, C., Wang, Y., Ye, W.,
Zhang, Y., Chang, Y., Yu, P. S., Yang, Q., and Xie,
X. (2024). A survey on evaluation of large language
models. ACM Transactions on Intelligent Systems and
Technology, 15.
Di Gennaro, L., de Cristofaro, M., Loreto, G., Minutolo, V.,
Olivares, L., Zona, R., and Frunzio, G. (2024). In-
situ load testing of an ancient masonry structure using
fibre optics. Structures, 70:107567.
Frumkin, M. (1947). Early history of patents for innovation.
Transactions of the Newcomen Society, 26.
Gennaro, L. D., Damiano, E., Cristofaro, M. D., Netti,
N., Olivares, L., Zona, R., Iavazzo, L., Coscetta, A.,
Mirabile, M., Giarrusso, G., D’Ettore, A., and Min-
utolo, V. (2022). An innovative geotechnical and
structural monitoring system based on the use of nsht.
Smart Materials and Structures, 31.
Jiang, S., Sarica, S., Song, B., Hu, J., and Luo, J. (2022).
Patent data for engineering design: A critical review
and future directions. Journal of Computing and In-
formation Science in Engineering, 22.
Just, J. (2024). Natural language processing for innovation
search – reviewing an emerging non-human innova-
tion intermediary. Technovation, 129.
Katz, D. M., Hartung, D., Gerlach, L., Jana, A., and Bom-
marito, M. J. (2023). Natural language processing in
the legal domain. SSRN Electronic Journal.
Krestel, R., Chikkamath, R., Hewel, C., and Risch, J.
(2021). A survey on deep learning for patent analy-
sis. World Patent Information, 65.
Mikolov, T., Chen, K., Corrado, G., and Dean, J. (2013).
Efficient estimation of word representations in vector
space. In 1st International Conference on Learning
Representations, ICLR 2013 - Workshop Track Pro-
ceedings.
Min, B., Ross, H., Sulem, E., Veyseh, A. P. B., Nguyen,
T. H., Sainz, O., Agirre, E., Heintz, I., and Roth, D.
(2023). Recent advances in natural language process-
ing via large pre-trained language models: A survey.
ACM Computing Surveys, 56.
Minutolo, V., Cerri, E., Coscetta, A., Damiano, E., Cristo-
faro, M. D., Gennaro, L. D., Esposito, L., Ferla,
P., Mirabile, M., Olivares, L., and Zona, R. (2020).
Nsht: New smart hybrid transducer for structural and
geotechnical applications. Applied Sciences (Switzer-
land), 10.
Radford, A. (2018). Improving language understanding
by generative pre-training. Homology, Homotopy and
Applications, 9.
Shalaby, W. and Zadrozny, W. (2019). Patent retrieval: a
literature review.
Vaswani, A., Shazeer, N., Parmar, N., Uszkoreit, J., Jones,
L., Gomez, A. N., Łukasz Kaiser, and Polosukhin,
I. (2017). Attention is all you need. In Advances
in Neural Information Processing Systems, volume
2017-December.
AI4EIoT 2025 - Special Session on Artificial Intelligence for Emerging IoT Systems: Open Challenges and Novel Perspectives
508