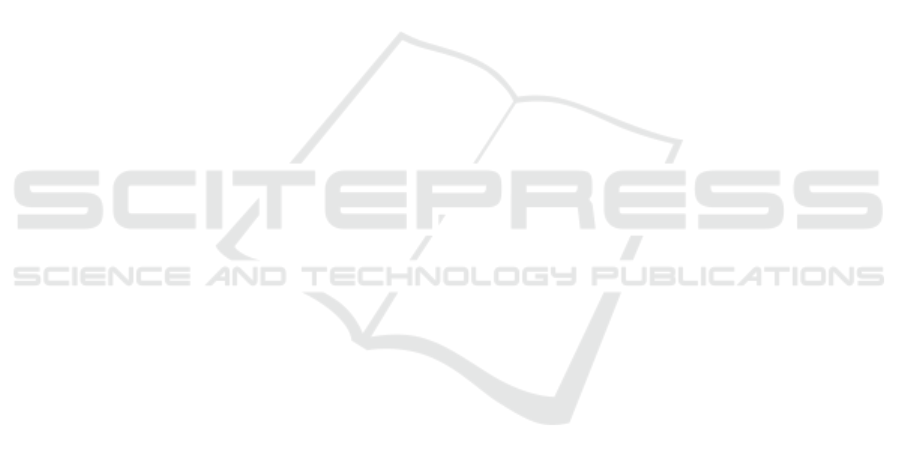
sis. Biomechanics and modeling in mechanobiology,
20(2):449–465.
Coorey, G., Figtree, G. A., Fletcher, D. F., Snelson, V. J.,
Vernon, S. T., Winlaw, D., Grieve, S. M., McEwan,
A., Yang, J. Y. H., Qian, P., et al. (2022). The health
digital twin to tackle cardiovascular disease—a review
of an emerging interdisciplinary field. NPJ digital
medicine, 5(1):126.
De Fazio, R., Nespolino, C., Marrone, S., and Verde, L.
(2025). A unified approach for digital twins in health-
care. In Handbook of Artificial Intelligence in Health-
care, under review.
Garc
´
ıa-Moll
´
a, V. M., Liberos, A., Vidal, A., Guillem, M.,
Millet, J., Gonzalez, A., Mart
´
ınez-Zald
´
ıvar, F.-J., and
Climent, A. M. (2014). Adaptive step ode algorithms
for the 3d simulation of electric heart activity with
graphics processing units. Computers in biology and
medicine, 44:15–26.
Hassani, H., Huang, X., and MacFeely, S. (2022). Impactful
Digital Twin in the Healthcare Revolution. Big Data
and Cognitive Computing, 6(3):83.
Hemamalini, V., Armosh, F., and Tyagi, A. K. (2024). Digi-
tal Twin-Based Smart Healthcare Services for the Next
Generation Society, page 247–277. IGI Global.
Hermida, U., van Poppel, M. P., Sabry, M., Keramati,
H., Steinweg, J. K., Simpson, J. M., Vigneswaran,
T. V., Razavi, R., Pushparajah, K., Lloyd, D. F.,
et al. (2024). The onset of coarctation of the aorta
before birth: Mechanistic insights from fetal arch
anatomy and haemodynamics. Computers in Biology
and Medicine, 182:109077.
Lagana, K., Balossino, R., Migliavacca, F., Pennati, G.,
Bove, E. L., de Leval, M. R., and Dubini, G. (2005).
Multiscale modeling of the cardiovascular system: ap-
plication to the study of pulmonary and coronary per-
fusions in the univentricular circulation. Journal of
biomechanics, 38(5):1129–1141.
Linial, O., Ravid, N., Eytan, D., and Shalit, U. (2021). Gen-
erative ODE modeling with known unknowns. In Pro-
ceedings of the Conference on Health, Inference, and
Learning, pages 79–94.
Marques, L., Costa, B., Pereira, M., Silva, A., Santos, J.,
Saldanha, L., Silva, I., Magalh
˜
aes, P., Schmidt, S.,
and Vale, N. (2024). Advancing precision medicine:
A review of innovative in silico approaches for drug
development, clinical pharmacology and personalized
healthcare. Pharmaceutics, 16(3):332.
Marrone, S. (2024). What does a heart beat for? a heteroge-
neous approach for human digital twin construction.
Procedia Computer Science, 246:5132–5141.
Mulder, S. T., Omidvari, A.-H., Rueten-Budde, A. J.,
Huang, P.-H., Kim, K.-H., Bais, B., Rousian, M., Hai,
R., Akgun, C., van Lennep, J. R., et al. (2022). Dy-
namic digital twin: Diagnosis, treatment, prediction,
and prevention of disease during the life course. Jour-
nal of Medical Internet Research, 24(9):e35675.
Okegbile, S. D., Cai, J., Niyato, D., and Yi, C. (2023).
Human Digital Twin for Personalized Healthcare: Vi-
sion, Architecture and Future Directions. IEEE Net-
work, 37(2):262–269.
Quarteroni, A., Manzoni, A., and Vergara, C. (2017). The
cardiovascular system: mathematical modelling, nu-
merical algorithms and clinical applications. Acta Nu-
merica, 26:365–590.
Ramasamy, L. K., Khan, F., Shah, M., Prasad, B. V. V. S.,
Iwendi, C., and Biamba, C. (2022). Secure smart
wearable computing through artificial intelligence-
enabled internet of things and cyber-physical systems
for health monitoring. Sensors, 22(3):1076.
Romero, P., Pedr
´
os, A., Sebastian, R., Lozano, M., and
Garc
´
ıa-Fern
´
andez, I. (2025). A robust shape model
for blood vessels analysis. Applied Mathematics and
Computation, 487:129078.
Salvador, M., Strocchi, M., Regazzoni, F., Augustin, C. M.,
Dede’, L., Niederer, S. A., and Quarteroni, A. (2024).
Whole-heart electromechanical simulations using la-
tent neural ordinary differential equations. NPJ Digi-
tal Medicine, 7(1):90.
Tanade, C., Khan, N. S., Rakestraw, E., Ladd, W. D.,
Draeger, E. W., and Randles, A. (2024). Establish-
ing the longitudinal hemodynamic mapping frame-
work for wearable-driven coronary digital twins. NPJ
Digital Medicine, 7(1):236.
Wang, B., Zhou, H., Yang, G., Li, X., and Yang, H.
(2022). Human digital twin (HDT) driven human-
cyber-physical systems: Key technologies and appli-
cations. Chinese Journal of Mechanical Engineering,
35(1):11.
Yu, W., Zhao, G., Liu, Q., and Song, Y. (2021). Role of
big data analytics capability in developing integrated
hospital supply chains and operational flexibility: An
organizational information processing theory perspec-
tive. Technological Forecasting and Social Change,
163:120417.
Zenker, S., Rubin, J., and Clermont, G. (2007). From in-
verse problems in mathematical physiology to quanti-
tative differential diagnoses. PLoS computational bi-
ology, 3(11):e204.
AI4EIoT 2025 - Special Session on Artificial Intelligence for Emerging IoT Systems: Open Challenges and Novel Perspectives
490