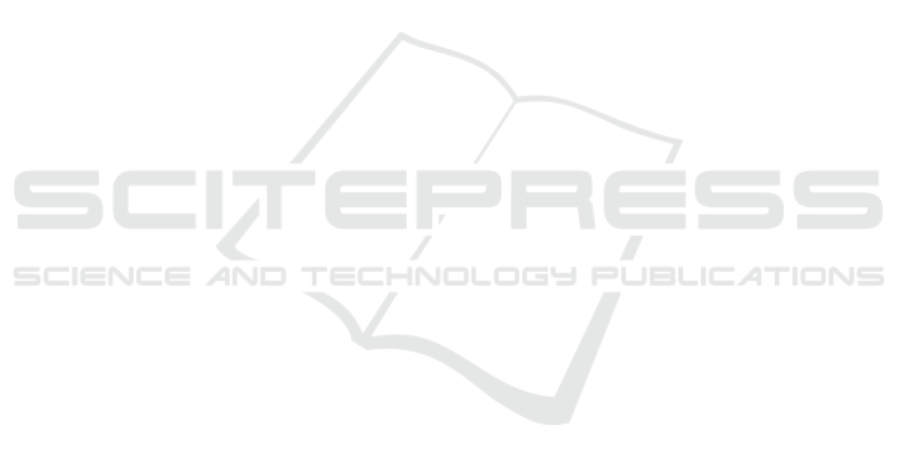
6.1 Future Directions
This study opens several promising avenues to en-
hance the effectiveness and practical applicability of
QCNNs. Incorporating noise mitigation strategies, in-
cluding quantum error mitigation and correction tech-
niques, could help to bridge the performance gap be-
tween noise-free and noisy models. Optimizing the
quantum circuit architecture by reducing the number
of entangling CNOT gates or by employing more ro-
bust quantum feature maps may further improve re-
silience against hardware imperfections. Expanding
the current binary classification framework to multi-
class scenarios would provide a more comprehensive
assessment of scalability and generalization. Finally,
validating QCNNs on real quantum hardware is es-
sential for directly assessing noise effects and mak-
ing realistic comparisons with classical CNNs. In
summary, while this study confirms the feasibility of
QCNNs for image classification, further advances in
error correction, hardware performance, and training
strategies are critical to fully harnessing the potential
of quantum neural networks.
ACKNOWLEDGEMENTS
This work was funded bythe European Union
- NextGenerationEU under the project NRRP
(i)“National Centre for HPC, Big Data and Quan-
tum Computing (HPC)” CN00000013 (CUP
D43C22001240001) [MUR Decree n. 1031-
17/06/2022] - Cascade Call launched by SPOKE
10 POLIMI: “QML-NTED” project, (ii) “National
Quantum Science & Technology Institute (NQSTI)”
PE00000023 (CUP B53C22004180005) [MUR
Decree n. 341 15/03/2022] – Cascade Call launched
by SPOKE 8 CNR: “QUANTIC” project. EU-
FESR, PON Ricerca e Innovazione 2014-2020-DM
1062/2021. The experiments have been performed
by using the computing resources operated by the
Department of Mathematics and Physics of the
University of Campania “Luigi Vanvitelli”, Caserta,
Italy, within the VALERE Program.
REFERENCES
Chen, G., Chen, Q., Long, S., Zhu, W., Yuan, Z., and Wu,
Y. (2023). Quantum convolutional neural network for
image classification. Pattern Analysis and Applica-
tions, 26(2):655 – 667.
Easom-Mccaldin, P., Bouridane, A., Belatreche, A., Jiang,
R., and Al-Maadeed, S. (2024). Efficient quantum im-
age classification using single qubit encoding. IEEE
Transactions on Neural Networks and Learning Sys-
tems, 35(2):1472 – 1486.
Gong, L.-H., Pei, J.-J., Zhang, T.-F., and Zhou, N.-R.
(2024). Quantum convolutional neural network based
on variational quantum circuits. Optics Communica-
tions, 550.
Hassan, E., Hossain, M. S., Saber, A., Elmougy, S.,
Ghoneim, A., and Muhammad, G. (2024). A quan-
tum convolutional network and resnet (50)-based clas-
sification architecture for the MNIST medical dataset.
Biomedical Signal Processing and Control, 87.
Hilbert, M. and L
´
opez, P. (2011). The world’s technolog-
ical capacity to store, communicate, and compute in-
formation. Science, 332(6025):60–65.
Huang, G. B., Mattar, M., Berg, T., and Learned-Miller,
E. (2008). Labeled faces in the wild: A database
forstudying face recognition in unconstrained envi-
ronments. In Workshop on faces in’Real-Life’Images:
detection, alignment, and recognition.
Huang, S.-Y., An, W.-J., Zhang, D.-S., and Zhou, N.-R.
(2023). Image classification and adversarial robust-
ness analysis based on hybrid quantum–classical con-
volutional neural network. Optics Communications,
533.
Kharsa, R., Bouridane, A., and Amira, A. (2023). Advances
in quantum machine learning and deep learning for
image classification: A survey. Neurocomputing, 560.
Lazzarin, M., Galli, D. E., and Prati, E. (2022). Multi-
class quantum classifiers with tensor network circuits
for quantum phase recognition. Physics Letters A,
434:128056.
Lu, Y., Gao, Q., Lu, J., Ogorzalek, M., and Zheng, J. (2021).
A quantum convolutional neural network for image
classification. volume 2021-July, page 6329 – 6334.
Oh, S., Choi, J., Kim, J.-K., and Kim, J. (2021). Quantum
convolutional neural network for resource-efficient
image classification: A quantum random access mem-
ory (QRAM) approach. volume 2021-January, page
50 – 52.
Schuld, M. and Petruccione, F. (2021). Machine learning
with quantum computers, volume 676. Springer.
Sebastianelli, A., Zaidenberg, D. A., Spiller, D., Le Saux,
B., and Ullo, S. (2022). On circuit-based hybrid quan-
tum neural networks for remote sensing imagery clas-
sification. IEEE Journal of Selected Topics in Ap-
plied Earth Observations and Remote Sensing, 15:565
– 580.
Wall, M. L. and D’Aguanno, G. (2021). Tree-tensor-
network classifiers for machine learning: From quan-
tum inspired to quantum assisted. Physical Review A,
104(4):042408.
Zhang, Z., Mi, X., Yang, J., Wei, X., Liu, Y., Yan, J., Liu,
P., Gu, X., and Yu, T. (2023). Remote sensing im-
age scene classification in hybrid classical–quantum
transferring cnn with small samples. Sensors, 23(18).
AI4EIoT 2025 - Special Session on Artificial Intelligence for Emerging IoT Systems: Open Challenges and Novel Perspectives
516